The Role of Computers in Finance: A Comprehensive Exploration
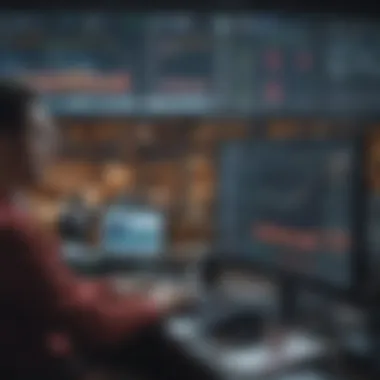
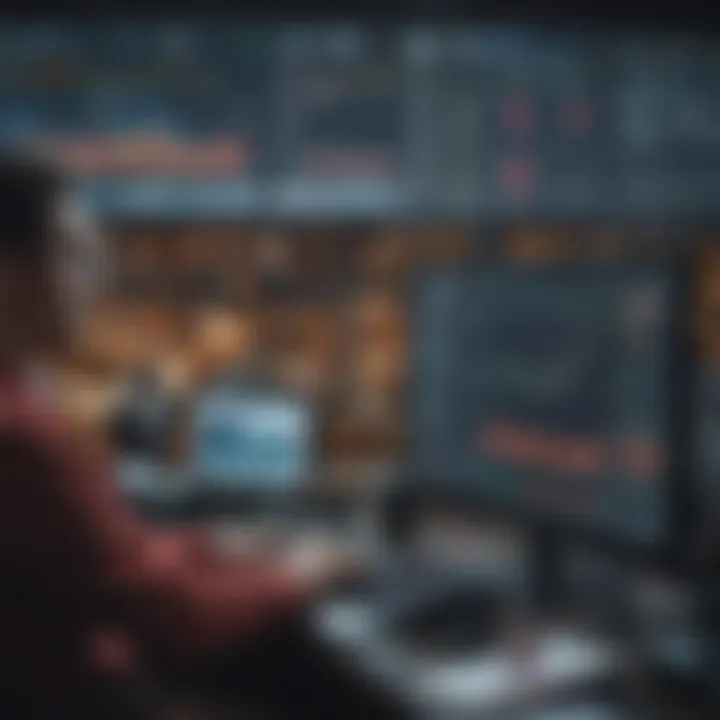
Intro
In the modern world, computers play a pivotal role in reshaping finance. The financial sector evolves rapidly, and amid this transformation, technology serves as the backbone of innovative practices and strategies. Gone are the days of simple ledgers and manual calculations; today's finance harnesses the power of computers to propel decision-making, enhance accuracy, and improve efficiency. This evolution opens up avenues for not just traditional investors, but also newcomers in the realm of cryptocurrency and blockchain technology.
As technology advances, keeping pace becomes essential. Let's explore the key concepts that define the fusion of finance and computing, delving into the indispensable tools that frame our financial environment. Understanding these aspects will help investors, analysts, and enthusiasts navigate the complexities of an ever-evolving market.
Prelims to Computers in Finance
In today’s whirlwind financial landscape, the integration of computers into finance isn't just a luxury; it's a necessity that's changed the game entirely. Understanding the nuances of how computers influence financial systems is essential for anyone who wishes to stay afloat in this fast-paced environment. This section will delve into why the marriage between technology and finance matters so much, exploring its implications for investors, analysts, educators, and developers alike.
The significance of computers in finance cannot be overstated. They provide the backbone for every transaction, enabling high-speed processing and data analysis that humans simply couldn't achieve alone. With the vast oceans of data generated every second, leveraging computational power is now central to informing decisions, mitigating risks, and ensuring compliance. Therefore, every stakeholder in the financial sector must grasp the landscape of financial computing.
The Evolution of Financial Computing
A look back reveals a fascinating evolution of financial computing—like watching a caterpillar morph into a butterfly. It all kicked off with basic calculations done on mechanical devices before transitioning to electronic calculators in the mid-20th century. Fast forward a few decades, and here we are, relying on sophisticated algorithms and artificial intelligence to manage investments, execute trades, and analyze market trends.
Regional markets that once depended solely on human intuition and manual entries have made room for syntactic precision and speed. The introduction of personal computers in the '80s was a game-changer, allowing individual investors to access and analyze data in real time instead of waiting for newspaper stock listings. Today, we are in an era where accessing financial information takes mere seconds, thanks to the internet and advanced computational techniques.
Furthermore, the proliferation of mobile technology has democratized finance, enabling an entire generation of retail investors to participate in markets that were once exclusive to institutional players. Companies now use sophisticated tools such as sentiment analysis algorithms that scour social media for market sentiment—a real twist compared to the old days of just waiting for quarterly earnings reports.
Significance of Computerization in Financial Markets
The computerization of financial markets ushers in a plethora of benefits—such as efficiency, speed, and accuracy—that were previously unimaginable.
- Efficiency: Automated processes streamline operations, reducing the time necessary to clear transactions from days to minutes, if not seconds.
- Speed: High-frequency trading firms execute thousands of trades in the blink of an eye, capitalizing on minute price fluctuations and generating profits that require a significant human touch in traditional methods.
- Accuracy: Computational tools minimize human error, safeguarding transactions and providing a more reliable environment for financial engagements.
"The efficiency achieved by deploying computers in finance has changed how we approach trading and risk management on an unprecedented scale."
Moreover, the surge of data analytics has made it possible for businesses to make informed decisions quicker than ever. Employing advanced analytics helps organizations tap into market trends, enhancing their competitive edge.
The computerization of finance isn't without its challenges; however, it brings new questions about security, ethics, and market volatility. Still, recognizing its benefits and understanding how to navigate these challenges is vital for today's finance professionals. As the landscape continues to evolve, those who can harness computational power in strategic ways stand to thrive.
Algorithmic Trading: The Intersection of Finance and Technology
Algorithmic trading stands at the crossroads of finance and cutting-edge technology. It harnesses advanced computational techniques to execute trades at speeds and volumes that humans simply cannot match. As we delve into this section, we will explore the key components of algorithmic trading, from various strategies employed to the impacts of speed and efficiency, along with the regulatory landscape that shapes it.
Overview of Algorithmic Trading Strategies
Algorithmic trading encompasses a myriad of strategies tailored for different market environments and objectives. Here, a few notable approaches are highlighted:
- Trend Following: Investors using this tactic look to capitalize on sustained market movements. Algorithms spot upward or downward trends and execute trades along the trajectory.
- Arbitrage Opportunities: This method focuses on price discrepancies between different markets or instruments. When an anomaly occurs, algorithms quickly trade the mispriced assets to realize immediate profits.
- Market Making: Here, algorithms provide liquidity to the market by continuously buying and selling assets, capturing the bid-ask spread as profit.
- Mean Reversion: This strategy is built on the assumption that prices will return to their historical average. When assets are overpriced or underpriced, the algorithm triggers trades to profit from the expected correction.
Each of these strategies necessitates a keen understanding of market behavior and advanced data analysis. By implementing these methods, traders aim to achieve consistent returns and mitigate risks.
The Impact of Speed and Efficiency
In the fast-paced world of financial trading, speed is often the name of the game. Algorithmic trading has drastically reduced the time it takes to execute trades—often within milliseconds. This speed brings several benefits:
- Increased Market Liquidity: With algorithms executing trades continuously, market depth increases, allowing other traders to benefit from more stable prices.
- Enhanced Precision: Algorithms can make calculations and decisions without the errors that might occur in human judgement resulting from emotional factors, fatigue, or stress.
- Cost Reduction: Automated trading processes can lower transaction costs. By executing trades at optimal times, they minimize slippage and market impact.
However, this lightning speed also presents challenges, such as the potential for flash crashes—events where prices plunge uncontrollably before rebounding. The reliance on technology leads to questions about market stability and the need for risk controls.
Regulatory Environment and Compliance
With the proliferation of algorithmic trading comes an evolving regulatory framework designed to ensure market integrity and protect investors. Regulations vary widely across jurisdictions but generally focus on:
- Market Surveillance: Regulators employ advanced monitoring systems to track trading activities. These systems are essential for identifying potential manipulative behaviors, like spoofing or layering.
- Compliance Measures: Trading firms must adhere to strict guidelines regarding the algorithms they deploy. This includes pre-trade risk checks, to prevent erroneous order placements that could disrupt markets.
- Transparency Requirements: Many jurisdictions require firms to disclose their trading strategies and ensure that their algorithms do not exploit confidential information or unequal access to market data.
"In the complex world of finance, proper regulation ensures that technology serves to enhance, not destabilize, the market environment."
Adherence to these regulations is paramount; failure to comply can lead to severe penalties, including fines and restriction from market participation.
Computational Finance: Tools and Techniques
In the realm of finance, the marriage of computation and analysis has opened doors to intricate solutions that shape the way we understand and navigate markets. Computational finance is not just a buzzword; it represents a crucial cog in the financial machinery, particularly in risk assessment, asset pricing, and portfolio management. This section delves into the essential tools and techniques that characterize this fascinating domain. It emphasizes the importance of mathematical models, statistical analysis, and risk management strategies in informing decision-making and optimizing performance.
Mathematical Models in Finance
Mathematical models serve as the backbone of computational finance, enabling professionals to simulate real-world scenarios and forecast potential outcomes. These models apply complex algorithms rooted in calculus and linear algebra to quantify uncertainties and probabilities associated with financial assets. By employing models such as the Black-Scholes for options pricing or the Capital Asset Pricing Model (CAPM) for risk assessment, investors can gauge expected returns under various market conditions.
Moreover, these models evolve with the financial landscape, accommodating new variables and data inputs. For instance, during economic volatility, multi-factor models incorporate aspects like macroeconomic indicators and investor sentiment to enhance predictive power. By utilizing these frameworks, analysts can approach investments with a critical eye, assessing the likelihood of success while mitigating potential losses.
Statistical Analysis and Its Applications
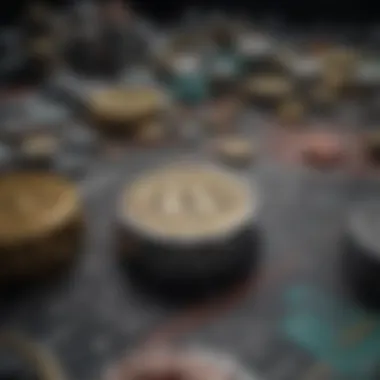
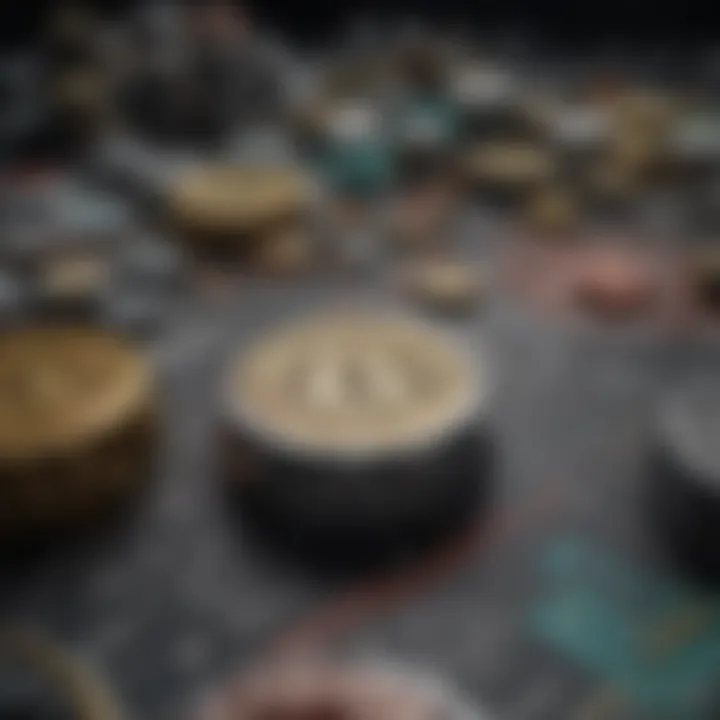
Statistical analysis plays an equally vital role in the armory of computational finance. With an abundance of data generated daily from various market interactions, the importance of extracting actionable insights cannot be overstated. Statistical techniques enable finance professionals to identify trends, correlations, and anomalies that might otherwise go unnoticed.
Through methodologies like regression analysis, analysts can predict market behaviors based on historical data. For example, by examining past price movements or trading volumes, professionals can construct probabilistic models that aid in forecasting future performance. Additionally, concepts like time-series analysis become particularly significant; they assist analysts in understanding price movements over time, accounting for seasonal variations and potential outliers.
- Common statistical tools and techniques include:
- Regression Analysis
- Time-Series Analysis
- Monte Carlo Simulations
These techniques allow for a nuanced approach to investment analysis, enabling professionals to make more informed decisions based on empirical data rather than gut feelings.
Risk Management through Computation
Risk management is arguably the centerpiece of computational finance. In an unpredictable market environment, the ability to model and manage risk is paramount for ensuring financial stability and achieving investment goals. Computational tools help finance professionals deploy risk assessment models that quantify the various risks associated with investments.
Techniques such as Value at Risk (VaR), stress testing, and scenario analysis are common in this arena. By evaluating potential losses in adverse market conditions or understanding the impact of extreme events, firms can adopt more robust strategies to protect their investments and assets.
- Key components of risk management include:
- Market Risk: Exposure to fluctuations in market prices
- Credit Risk: The risk of default on obligations
- Operational Risk: Potential losses from inadequate systems or processes
Data Analytics in Finance
Data analytics has become a cornerstone in the contemporary financial landscape, marking a shift in how data is utilized to inform decisions, uncover trends, and predict future movements. In the context of this article, understanding the evolving role of data analytics in finance is essential. This section provides a framework to comprehend how big data, predictive analytics, and consumer behavior analysis come together to drive the financial sector forward.
Big Data and Financial Decision Making
In the realm of finance, big data isn't just a buzzword; it’s the lifeblood that fuels strategic decision-making. With vast amounts of data generated daily—from market transactions to social media interactions—financial institutions must harness this information effectively.
The benefits of big data are manifold:
- Enhanced Predictive Power: By analyzing historical trends, financial organizations can better forecast future performance, allowing for informed investments and risk assessments.
- Operational Efficiency: Automation powered by big data analytics helps streamline processes, thus reducing costs and improving service delivery.
- Personalized Financial Services: With insights gleaned from big data, firms can tailor their products to meet individual customer needs, enhancing customer satisfaction and loyalty.
Yet, potential challenges exist. Issues like data privacy, quality control, and integration with existing systems complicate the picture. Properly addressing these considerations can mean the difference between leveraging big data effectively or wasting resources.
Predictive Analytics and Market Trends
Predictive analytics rises as a powerful tool that utilizes statistical algorithms and machine learning techniques to identify the likelihood of future outcomes based on historical data. When it comes to financial markets, predictive analytics can significantly impact:
- Market Analysis: By identifying patterns, investors and analysts can discern potential market trends, enhancing forecasting accuracy.
- Risk Assessment: Assessing credit risk and potential defaults becomes more precise when backed by robust predictive models.
- Resource Allocation: Financial institutions can optimize their investment strategies, directing funds toward assets with the highest yield potential.
The use of advanced models—such as regression analysis and neural networks—enables firms to make sense of complex datasets, providing a competitive edge. However, relying solely on predictive analytics carries risks. External factors, such as geopolitical events or crises, can drastically affect market dynamics, making it vital only to use predictive analytics as one piece of a larger puzzle.
Consumer Behavior Analysis
Understanding consumer behavior adds another layer to the financial decision-making process. As customers increasingly interact with financial products through digital platforms, their behavior offers valuable insights into preferences and trends. Financial institutions need to focus on:
- Engagement Metrics: Tracking how clients are interacting with their services can reveal loopholes and opportunities for improvement.
- Sentiment Analysis: Analyzing consumer sentiment—especially online—provides insight into brand reputation and customer trust, affecting investment decisions.
- Customized Products: By understanding consumer preferences, companies can devise targeted marketing strategies, potentially increasing conversion rates and retention.
Nonetheless, privacy concerns arise as more data is collected. It’s crucial for firms to maintain ethical standards while leveraging consumer insights. Laws governing data protection should not only be adhered to but embraced as a core principle of responsible data analytics.
"Data is the new oil. It’s valuable, but if unrefined, it cannot really be used. Data must be broken down, analyzed for it to have any meaningful value in finance."
To sum up, integrating data analytics into financial strategies isn’t merely beneficial; it’s imperative. As organizations grow their analytical capacities, they must navigate challenges carefully to harness the full potential of data-driven finance.
Artificial Intelligence and Machine Learning in Finance
Artificial Intelligence (AI) and Machine Learning (ML) are powerful drivers of change in the finance sector. The growing importance of these technologies cannot be overstated, as they equip financial institutions with the tools needed to analyze massive datasets, predict trends, and improve decision-making processes. For investors, analysts, and developers, understanding the nuances of AI and ML applications in finance is crucial, as it directly influences the efficiency and effectiveness of financial operations.
AI technologies enhance strategies in wealth management, risk assessment, and fraud detection, among others. By harnessing the computational power of AI, financial organizations can offer personalized advice, streamline operations, and safeguard against fraud.
AI Applications in Wealth Management
In wealth management, AI systems analyze client behavior, financial goals, and market conditions to provide tailored investment advice. These systems use complex algorithms to process historical data and forecast potential returns, thereby allowing advisors to optimize client portfolios. For instance, robo-advisors like Betterment utilize AI to manage investments automatically, adapting strategies based on changing market conditions while considering client risk tolerances. This not only reduces costs associated with traditional advisors but also democratizes wealth management by making it accessible to a broader audience.
Furthermore, the use of natural language processing enables these systems to decipher market sentiments from various data sources, enhancing decision-making capabilities.
Credit Scoring and Risk Assessment
In credit scoring, AI adds a layer of complexity and accuracy. Traditional scoring models rely on a limited number of indicators, often leading to biased assessments. However, AI can sift through vast datasets, evaluating factors such as transaction history, payment behaviors, and even social media activity.
By leveraging ML algorithms, financial institutions can develop more comprehensive and fair credit scoring systems. These systems not only identify potential borrowers more reliably but also minimize the risks of defaults by assessing creditworthiness in a nuanced manner. The resulting models can adapt and improve over time, reshaping how lenders approach risk assessment.
Fraud Detection Systems
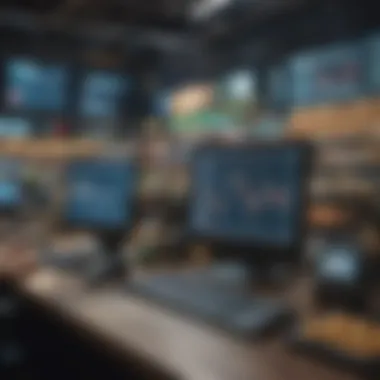
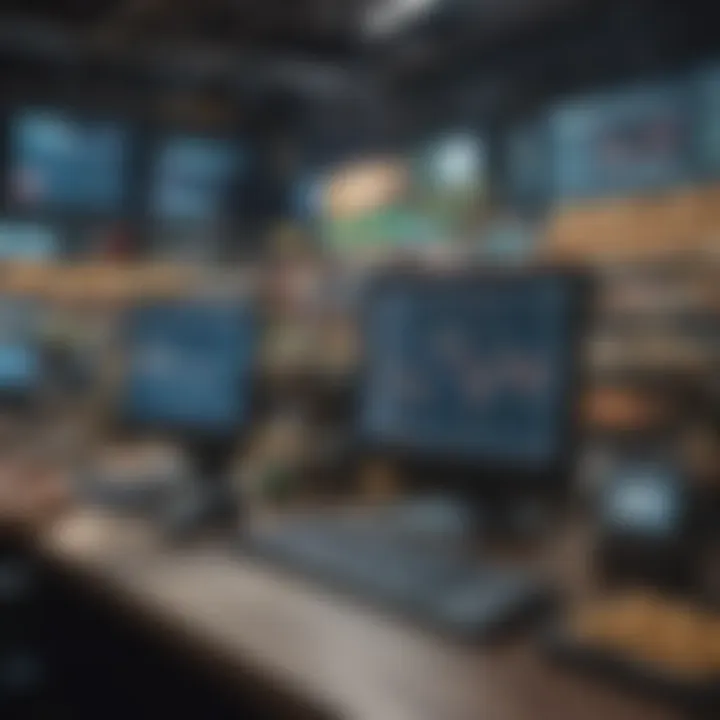
Fraud detection is another domain where AI shines. Financial institutions are prime targets for cybercriminals, making robust fraud prevention systems indispensable. Various AI-powered systems monitor transaction patterns in real-time, using algorithms trained on historical fraud data. This enables banks to identify anomalies that could signal fraudulent activity.
A notable example is PayPal’s use of machine learning to detect unusual user behavior. When it identifies a transaction that contradicts established patterns, the system triggers alerts, allowing for swift intervention. The efficiency of these systems not only protects consumers but also saves companies significant amounts of money by reducing fraud losses.
"A proactive approach to fraud detection, powered by AI, not only safeguards assets but also enhances consumer trust in financial institutions."
In summary, AI and ML are transforming finance by improving wealth management, refining credit scoring, and bolstering fraud detection strategies. Their rapid development aligns with the growing demands and challenges of the financial industry. For those looking to navigate this evolving landscape, understanding the potential and limitations of these technologies is essential.
Blockchain Technology and Its Financial Implications
The advent of blockchain technology has redefined many sectors, and finance stands at the forefront of this transformation. The integration of blockchain into financial systems not only enhances transparency but also significantly boosts the efficiency of transactions. This section delves into the foundational concepts of blockchain, its implications for distributed ledger technology, and the role of smart contracts in reshaping financial transactions.
Understanding Blockchain Fundamentals
At its core, blockchain is a decentralized digital ledger that securely records transactions across multiple computers. This technology ensures that once a transaction is recorded, it cannot be altered retroactively without altering all subsequent blocks, which is nearly impossible without consensus from the network. The key characteristics that make blockchain appealing to the financial sector include:
- Decentralization: Unlike traditional ledgers controlled by a central authority, blockchain operates on a peer-to-peer network, reducing the risk of centralized fraud.
- Immutability: Once data is recorded on a blockchain, it remains unchanged, providing an encryption-driven signature that guarantees authenticity and integrity.
- Transparency: Every participant in the network can access the same data, promoting trust among users and reducing disputes.
A significant takeaway for investors is that blockchain technology reduces the costs associated with transactions by removing intermediaries, streamlining processes, and increasing overall transaction speed.
Distributed Ledger Technology in Finance
Distributed Ledger Technology (DLT) is a critical aspect of blockchain that enables multiple parties to record, share, and synchronize their data in real-time. In finance, this technology finds its application in several areas:
- Settlements: DLT can expedite the settlement of trades by eliminating the time-consuming processes traditionally involved. For instance, cross-border payments, usually taking days, can now occur in minutes.
- Record Keeping: Financial institutions benefit from the enhanced accuracy and security that DLT offers. It can dramatically reduce errors and disputes over data discrepancies.
- Identity Verification: DLT can facilitate secure identity verification processes, significantly impacting KYC (Know Your Customer) practices in banking and finance.
Investors should be aware that while DLT presents numerous opportunities, it also poses challenges, such as regulatory uncertainties and technical integration with existing systems.
Smart Contracts: Revolutionizing Transactions
Smart contracts are self-executing contracts with the terms directly written into code. These contracts automatically execute actions when predefined conditions are met, greatly streamlining the process of contract management in finance. Here are some notable characteristics and benefits of smart contracts:
- Automation: Smart contracts minimize the need for intermediaries, thus expediting contract fulfillment while reducing operational costs.
- Accuracy: Given that smart contracts are based on coded terms, they minimize human error or misinterpretation, ensuring a higher level of precision in financial agreements.
- Trust and Security: The use of blockchain for smart contracts ensures that all parties involved can trust that terms will be executed without risk of tampering or dishonesty.
"Smart contracts represent a paradigm shift in how financial agreements are executed, increasing the safety and efficiency of the transactions dramatically."
The Role of Computers in Financial Regulation
In today's fast-paced financial sector, the role of computers stretches far beyond mere transactional calculations. They form the backbone of financial regulation, enhancing not only compliance but also fostering transparency and trust in the financial markets. As regulations become more stringent and complex, computers help financial institutions stay on the right side of the law while minimizing risks related to fraud and misconduct. The importance of leveraging technology in this field cannot be overstated. With the need for real-time data processing and monitoring, computers support regulators and firms in their critical operations.
Surveillance and Monitoring Systems
Central to financial regulation is the implementation of surveillance and monitoring systems. These systems are designed to detect suspicious activities and abnormalities in trading behaviors that may indicate market manipulation or insider trading. For instance, algorithms can analyze trading patterns by scanning vast amounts of data, identifying anomalies within milliseconds. This capability allows for the prompt addressing of potential violations before they escalate.
- Benefits of Surveillance Systems:
- Real-Time Monitoring: Continuous oversight means issues can be flagged immediately.
- Data Triangulation: Combines various data sources to provide clearer insights.
- Improved Accuracy: Reduces human errors through automated analysis.
While many of these systems are automated, it’s essential to combine technology with human oversight. Experts need to review flagged transactions to ensure appropriate actions are taken. Regulation is not just about technology; it’s about understanding operational nuances that algorithms might overlook. By focusing on both aspects, the financial sector can foster a safer trading environment.
Compliance Automation Tools
Another crucial element of computer use in regulation involves compliance automation tools. These tools help institutions manage their extensive regulatory obligations with efficiency and precision. In an era where the amount of regulatory requirements can feel like trying to drink from a fire hose, compliance automation offers a lifeline. This tech-centric approach allows firms to evaluate their adherence to laws more systematically.
Some notable features of these tools include:
- Automated Reporting: Streamlines the preparation and submission of required reports.
- Regulatory Change Tracking: Keeps organizations informed about evolving regulations.
- Centralized Data Management: Organizes compliance data in a single location for easy access.
The efficiency gained through automated compliance systems not only reduces the labor-intensive tasks associated with meeting regulatory demands but also lowers the risk of compliance breaches, which can lead to significant financial penalties. Furthermore, these tools can incorporate machine learning, which enhances their capabilities over time by learning from new data sets.
"In finance, the only constant is change. Automation is not just a trend; it's a necessity for survival."
As the landscape of financial regulation evolves, it’s clear that computers are not just supplementary; they are essential players in ensuring that the industry adheres to its legal responsibilities. Relying on sophisticated systems for both surveillance and compliance equips financial institutions to navigate the complexities of today's regulatory environment effectively.
Cybersecurity in Financial Computing
In an age where digital transactions are the norm, cybersecurity in financial computing isn’t just a nice-to-have; it's a must. With the rapid adoption of technology in finance, from banking apps to investment platforms, the risks associated with cyber threats have grown exponentially. The potential for data breaches, financial fraud, and system failures underscores the critical importance of cybersecurity measures that organizations must adopt to protect sensitive information. This section takes a closer look at the multifaceted threat landscape and how best to address these challenges.
Threat Landscape in Financial Technology
The threat landscape in financial technology has expanded and evolved, posing unique risks to institutions and individual stakeholders alike. Here are some key elements that define the current landscape:
- Phishing Attacks: Cybercriminals often employ phishing tactics, tricking employees into revealing sensitive credentials. This can lead to unauthorized access to financial systems.
- Malware: Malicious software can infiltrate financial systems, causing severe disruptions. Ransomware, in particular, has been on the rise, holding data hostage until a ransom is paid.
- Data Breaches: Financial institutions are prime targets for data breaches. Sensitive information such as account details, Social Security numbers, and credit card information is highly sought after by hackers.
- DDoS Attacks: Distributed Denial of Service (DDoS) attacks aim to overwhelm servers, making websites and online services unavailable. Such attacks can cripple banking operations and erode customer trust.
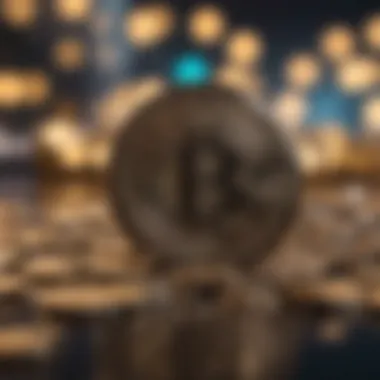
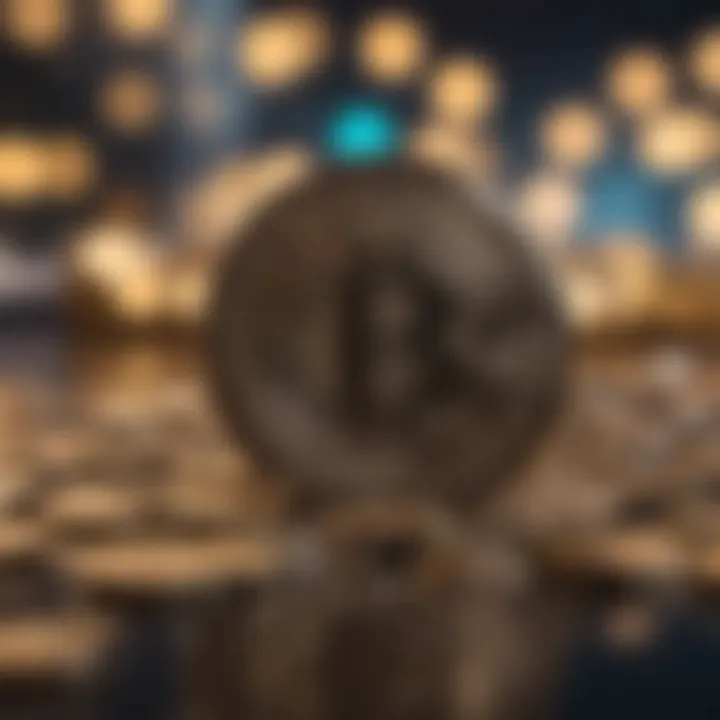
"In today's digital age, securing financial systems is not just about compliance but about maintaining trust with clients and stakeholders."
Organizations must stay alert to emerging threats and adjust their cybersecurity strategies accordingly. It's essential to recognize that the tactics used by cybercriminals continue to change, requiring constant vigilance and timely updates to security protocols.
Best Practices for Security in Finance
Adopting effective cybersecurity practices can significantly reduce vulnerability to financial threats. While no system is entirely invulnerable, certain strategies can enhance overall security posture:
- Regular Security Audits: Conducting thorough audits can help identify weaknesses in current systems. This should include external penetration testing to uncover vulnerabilities before they can be exploited.
- Employee Training: All members of an organization should receive training on identifying potential threats like phishing attempts. A well-informed team can act as the first line of defense against cyber threats.
- Data Encryption: Encrypting sensitive financial data can protect it from unauthorized access. Even if data is intercepted, encrypted information is much harder to decode.
- Multi-Factor Authentication (MFA): MFA adds an extra layer of security to the login process, making it significantly more difficult for unauthorized users to gain access.
- Incident Response Plans: Having a clear, actionable incident response plan can mitigate damage in the event of a cyber-attack. This involves having a step-by-step process for handling breaches and informing affected parties.
- Up-to-Date Software: Using the latest security software and keeping all systems updated is essential for patching vulnerabilities that criminals might exploit.
By implementing these best practices, organizations can better safeguard their financial systems. ?? As the financial landscape continues to intertwine with technology, the commitment to robust cybersecurity will determine not only compliance but also competitive advantage and customer trust.
Emerging Trends in Financial Computing
As we dive into the emerging trends in financial computing, it is essential to appreciate the colossal shifts occurring on this front. The fusion of technology with finance is not just from the sidelines; it’s a full-on game changer. Investors and analysts need to keep their fingers on the pulse of these trends to make informed decisions in an increasingly complex environment.
Decentralized Finance (DeFi) and Technology
Decentralized Finance, or DeFi, presents a fresh perspective on how transactions can be conducted without traditional intermediaries. Imagine cutting out the banks altogether—just you, your assets, and a peer-to-peer network. That's the power of DeFi. With platforms like Uniswap and Aave sprouting up, users can lend, borrow, and trade cryptocurrencies effortlessly.
One notable feature is the use of smart contracts, which automate processes and remove human error. They execute transactions automatically based on predefined conditions. This not only reduces costs but also speeds up transaction times. Moreover, by utilizing blockchain technology, DeFi enhances transparency, allowing anyone to scrutinize its transactions.
However, the rise of DeFi isn't without challenges. There are considerable risks, including smart contract vulnerabilities and regulatory uncertainties. Users must navigate these waters carefully. Investors looking to harness DeFi must be well-versed in the technology behind it and the potential risks involved.
The Future of FinTech Innovations
The trajectory of financial technology continues to climb at an unprecedented rate. In the next few years, we can expect to see innovations such as digital identities, advanced biometrics, and automated financial advisors more prevalent in the market. The shift towards digital currencies, like Central Bank Digital Currencies (CBDCs), is another pivotal trend. Countries are exploring CBDCs to enhance payment systems while retaining control over the monetary flow.
Furthermore, customer experience is evolving through technologies like machine learning and artificial intelligence. These technologies analyze consumer preferences, helping financial institutions tailor their services. Imagine getting investment advice uniquely suited to your spending habits and risk tolerance without consulting a human advisor— that's the kind of future we're heading into.
"Innovation in FinTech is not just a trend, it’s a necessity for survival in an ever-changing economic landscape."
In summary, these emerging trends are reshaping how we perceive and interact with finance. Understanding these developments is crucial for staying ahead, whether you are an investor or a tech enthusiast. Remaining adaptable and informed will be vital as the landscape continues to morph at breakneck speed.
Challenges in Implementing Computers in Finance
The integration of computers in finance is not without its hurdles. Organizations are constantly striving to incorporate advanced technology into their operations. However, challenges abound that can hinder progress and efficiency. Understanding these difficulties is crucial for anyone involved in the financial sector, whether they are seasoned investors, analysts, or enthusiastic developers.
Integration with Legacy Systems
One of the primary difficulties in modernizing financial systems is the presence of legacy systems. Most financial institutions have been using these old systems for decades, and they often form the backbone of operations. Switching to new computer solutions can feel like changing a tire while the car is still moving.
Key Issues:
- Data Compatibility: Legacy systems may store data in formats that are incompatible with newer technologies. This can lead to significant costs and delays in integrating software.
- Knowledge Gaps: There's often a gap in knowledge as team members familiar with legacy systems may not have the experience with newer technologies, complicating the overhaul.
- Resistance to Change: Employees may be hesitant to embrace new systems due to comfort with existing processes. This fear of change can stall implementation efforts.
Organizations must find a way to effectively navigate these roadblocks. It often requires a considerable investment of resources, both in money and time, to retrain staff and ensure seamless transitions.
Scalability and Flexibility Concerns
Another substantial challenge lies in scalability and flexibility. As financial markets become more complex and interconnected, the demand for computer systems that can efficiently scale is paramount. Companies need systems that not only meet current demands but can also grow with them.
Considerations:
- Current Infrastructure Limitations: Many traditional systems are not designed to handle rapidly increasing amounts of data and transactions. This makes them less effective in a high-frequency trading environment, where speed is everything.
- Future-Proofing Technology: Adopting flexible technologies that can adjust to changing market conditions is vital. However, this can pose a risk as businesses must constantly assess their technology to avoid obsolescence.
- Cost Implications: Upgrading systems for scalability can be expensive. Firms need to balance current financial constraints with long-term growth strategies, making decisions complicated.
The failure to address both integration and scalability issues can lead to inefficiencies. This is not just about having shiny new tech; it’s about having systems that bolster financial decision-making capabilities.
Effective use of technology in finance is integral to staying competitive. Firms must be willing to adapt and innovate, or risk being left in the dust.
By recognizing and addressing these challenges, those involved in finance can better position themselves for future success. The implementation of computers in finance is a multi-faceted process that requires meticulous planning and execution.
Closure
The conclusion serves as a critical juncture, weaving together the insights gleaned throughout the sections outlined earlier. As we've traversed the landscape of finance through the prism of computing, it’s become increasingly clear that the relationship between technology and finance is not merely an enhancement but a fundamental shift. This shift translates into improved accuracy, enhanced efficiency, and the capability to analyze vast swathes of data instantaneously—elements that are indispensable for modern-day investors and analysts.
The Future Landscape of Finance and Technology
Looking ahead, the trajectory of finance is carved out by technological innovation. We find ourselves amidst a period characterized by fast-paced advancements. These innovations aren't just about convenience; they fundamentally alter how capital flows, how risks are assessed, and how investments are strategized.
Some key trends that stand out include:
- Artificial Intelligence and Automation: As AI continues to mature, expect to see increased automation in trading processes and risk assessments, allowing for quicker and more informed decisions. A bank's shift towards AI could lead to reduced operational costs while enhancing performance.
- Decentralized Finance (DeFi): This emerging trend leverages blockchain technology to recreate traditional financial systems in a decentralized manner. The implications of DeFi for financial institutions and individual investors are profound, offering new opportunities and challenges.
- Consumer-Centric Financial Solutions: The rise of fintech companies continues to prioritize customer experience. Solutions are being tailored to address individual financial needs, enhancing accessibility for previously underserved segments of the population.
It’s imperative for stakeholders in the finance sector to stay agile and rethink their strategies in response to these trends. If technology has shown us anything, it’s that change is not only possible—it is inevitable.
Final Thoughts on Financial Computing
In reflection, financial computing is more than a tool; it's a lens to perceive the future of finance itself. The crux of financial computing lies in its ability to process information and draw insights from it effectively.
For investors, analysts, and educators alike, understanding the implications of advanced computing in finance becomes crucial. Leveraging tools like predictive analytics can aid in making informed investment decisions. Moreover, staying informed about cybersecurity practices ensures the protection of sensitive financial data in an ever-evolving threat landscape.